DeepMind's GNoME Paper GNoME Illuminates AI-Driven Materials Discovery, But Challenges Remain
DeepMind's GNoME Paper GNoME Illuminates AI-Driven Materials Discovery, But Challenges Remain
DeepMind's GNoME Paper GNoME Illuminates AI-Driven Materials Discovery, But Challenges Remain
October 3, 2024



The development of new materials is of paramount importance in addressing emerging challenges and meeting evolving needs, such as sustainability concerns and the demand for higher-performance materials. However, the process of developing these new materials is notoriously difficult. It involves balancing a multitude of constraints, such as cost, durability, and specific application requirements, which are often hard to simulate accurately. This complexity is particularly pronounced in high-stakes industries where the margin for error is minimal, making traditional methods of materials discovery—largely based on trial and error and extensive physical testing—both time-consuming and inefficient.
In recent years, artificial intelligence (AI) has emerged as a powerful tool to assist in scientific fields, such as drug discovery. However, AI was not much used in the materials discovery process. At Osium AI, we noticed this gap and therefore built our technology with the strong belief that AI can drive valuable insights, significantly accelerating the pace of materials innovation. Our conviction is that AI can not only enhance the ability to discover new materials but also help optimize the development processes, reducing both time and cost.
At Osium AI, we developed our proprietary AI-powered platform to accelerate materials development well before Google DeepMind’s release of the Graph Networks for Materials Exploration (GNoME) paper a few months ago. We were excited to see this paper highlight the benefits of AI for materials discovery, in alignment with our belief in its transformative potential and paving the way for further innovation in the field.
The release of the GNoME paper has sparked widespread discussions in the materials science field, making it natural for us to cover their research, highlight their achievements, and assess how the paper positions itself within the broader materials science industry. Despite its strong potential, GNoME approach lacks industrial proximity, not taking into account industrial constraints.
This is where Osium AI comes into play, bridging the gap between theoretical research and practical industrial applications.
The development of new materials is of paramount importance in addressing emerging challenges and meeting evolving needs, such as sustainability concerns and the demand for higher-performance materials. However, the process of developing these new materials is notoriously difficult. It involves balancing a multitude of constraints, such as cost, durability, and specific application requirements, which are often hard to simulate accurately. This complexity is particularly pronounced in high-stakes industries where the margin for error is minimal, making traditional methods of materials discovery—largely based on trial and error and extensive physical testing—both time-consuming and inefficient.
In recent years, artificial intelligence (AI) has emerged as a powerful tool to assist in scientific fields, such as drug discovery. However, AI was not much used in the materials discovery process. At Osium AI, we noticed this gap and therefore built our technology with the strong belief that AI can drive valuable insights, significantly accelerating the pace of materials innovation. Our conviction is that AI can not only enhance the ability to discover new materials but also help optimize the development processes, reducing both time and cost.
At Osium AI, we developed our proprietary AI-powered platform to accelerate materials development well before Google DeepMind’s release of the Graph Networks for Materials Exploration (GNoME) paper a few months ago. We were excited to see this paper highlight the benefits of AI for materials discovery, in alignment with our belief in its transformative potential and paving the way for further innovation in the field.
The release of the GNoME paper has sparked widespread discussions in the materials science field, making it natural for us to cover their research, highlight their achievements, and assess how the paper positions itself within the broader materials science industry. Despite its strong potential, GNoME approach lacks industrial proximity, not taking into account industrial constraints. This is where Osium AI comes into play, bridging the gap between theoretical research and practical industrial applications.
The development of new materials is of paramount importance in addressing emerging challenges and meeting evolving needs, such as sustainability concerns and the demand for higher-performance materials. However, the process of developing these new materials is notoriously difficult. It involves balancing a multitude of constraints, such as cost, durability, and specific application requirements, which are often hard to simulate accurately. This complexity is particularly pronounced in high-stakes industries where the margin for error is minimal, making traditional methods of materials discovery—largely based on trial and error and extensive physical testing—both time-consuming and inefficient.
In recent years, artificial intelligence (AI) has emerged as a powerful tool to assist in scientific fields, such as drug discovery. However, AI was not much used in the materials discovery process. At Osium AI, we noticed this gap and therefore built our technology with the strong belief that AI can drive valuable insights, significantly accelerating the pace of materials innovation. Our conviction is that AI can not only enhance the ability to discover new materials but also help optimize the development processes, reducing both time and cost.
At Osium AI, we developed our proprietary AI-powered platform to accelerate materials development well before Google DeepMind’s release of the Graph Networks for Materials Exploration (GNoME) paper a few months ago. We were excited to see this paper highlight the benefits of AI for materials discovery, in alignment with our belief in its transformative potential and paving the way for further innovation in the field.
The release of the GNoME paper has sparked widespread discussions in the materials science field, making it natural for us to cover their research, highlight their achievements, and assess how the paper positions itself within the broader materials science industry. Despite its strong potential, GNoME approach lacks industrial proximity, not taking into account industrial constraints. This is where Osium AI comes into play, bridging the gap between theoretical research and practical industrial applications.
GNoME research review
GNoME research review
GNoME research review
Summary of the outcomes
Summary of the outcomes
Summary of the outcomes
On the 29 November of 2023, Google Deepmind sparked unrest when releasing a paper saying that they discovered 2.2 million new inorganic crystals, among which 380.000 are the most stable materials. To do so, they developed a new deep learning tool named GNoME.
Why is it special?
This discovery is special because it is equivalent to 800 years’ worth of knowledge. Indeed, the graph below depicts the amount of materials that have been discovered thanks to GNoME compared to other methods so far:
About 20,000 of the crystals in the ICSD database were at first discovered thanks to human experimentation
Then, Computational approaches drawing from the Materials Project, Open Quantum Materials Database and WBM database boosted this number to 48,000 stable crystals.
GNoME expanded the number of stable materials known to humanity to 421,000.
On the 29 November of 2023, Google Deepmind sparked unrest when releasing a paper saying that they discovered 2.2 million new inorganic crystals, among which 380.000 are the most stable materials. To do so, they developed a new deep learning tool named GNoME.
Why is it special?
This discovery is special because it is equivalent to 800 years’ worth of knowledge. Indeed, the graph below depicts the amount of materials that have been discovered thanks to GNoME compared to other methods so far:
About 20,000 of the crystals in the ICSD database were at first discovered thanks to human experimentation
Then, Computational approaches drawing from the Materials Project, Open Quantum Materials Database and WBM database boosted this number to 48,000 stable crystals.
GNoME expanded the number of stable materials known to humanity to 421,000.
On the 29 November of 2023, Google Deepmind sparked unrest when releasing a paper saying that they discovered 2.2 million new inorganic crystals, among which 380.000 are the most stable materials. To do so, they developed a new deep learning tool named GNoME.
Why is it special?
This discovery is special because it is equivalent to 800 years’ worth of knowledge. Indeed, the graph below depicts the amount of materials that have been discovered thanks to GNoME compared to other methods so far:
About 20,000 of the crystals in the ICSD database were at first discovered thanks to human experimentation
Then, Computational approaches drawing from the Materials Project, Open Quantum Materials Database and WBM database boosted this number to 48,000 stable crystals.
GNoME expanded the number of stable materials known to humanity to 421,000.
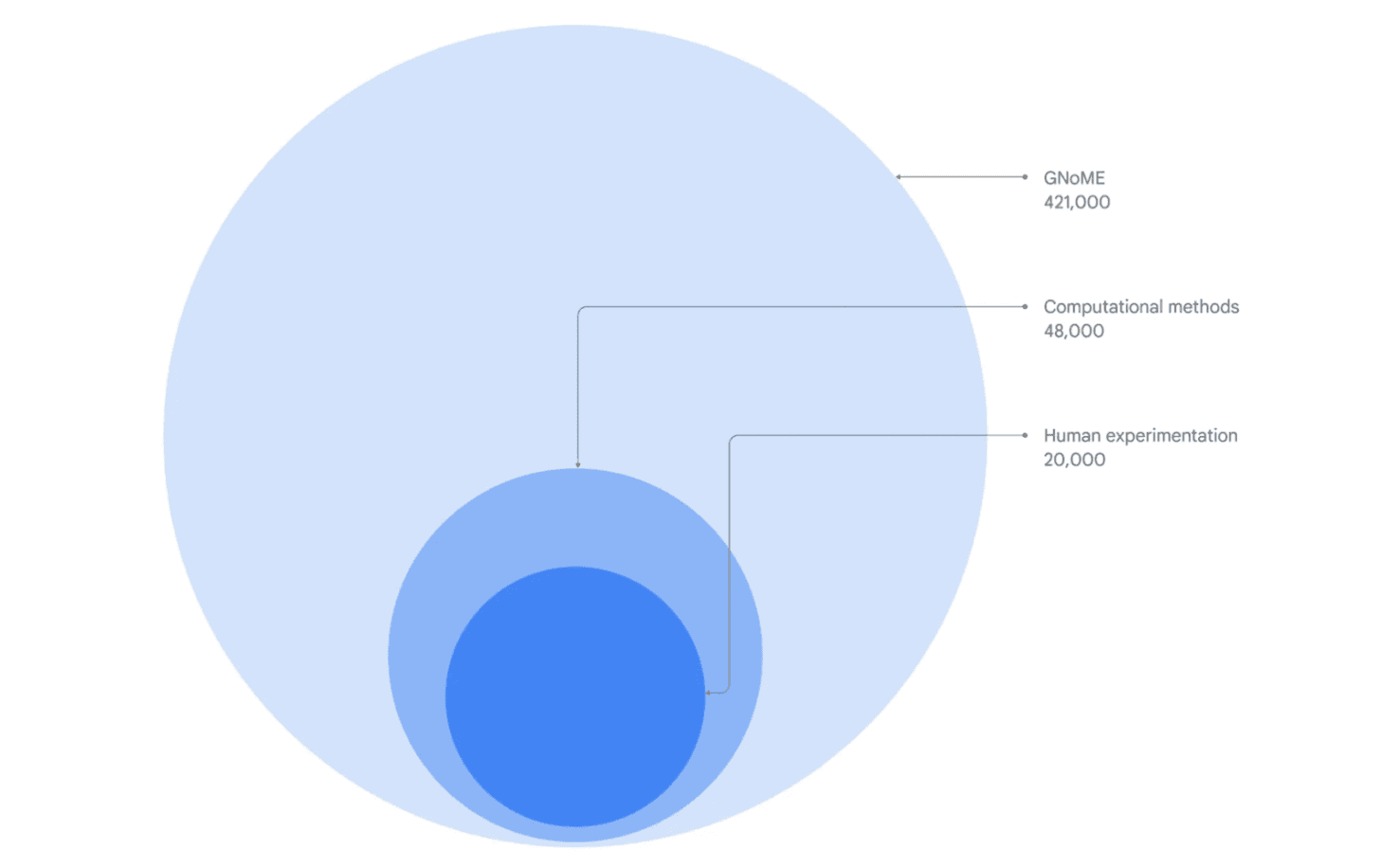
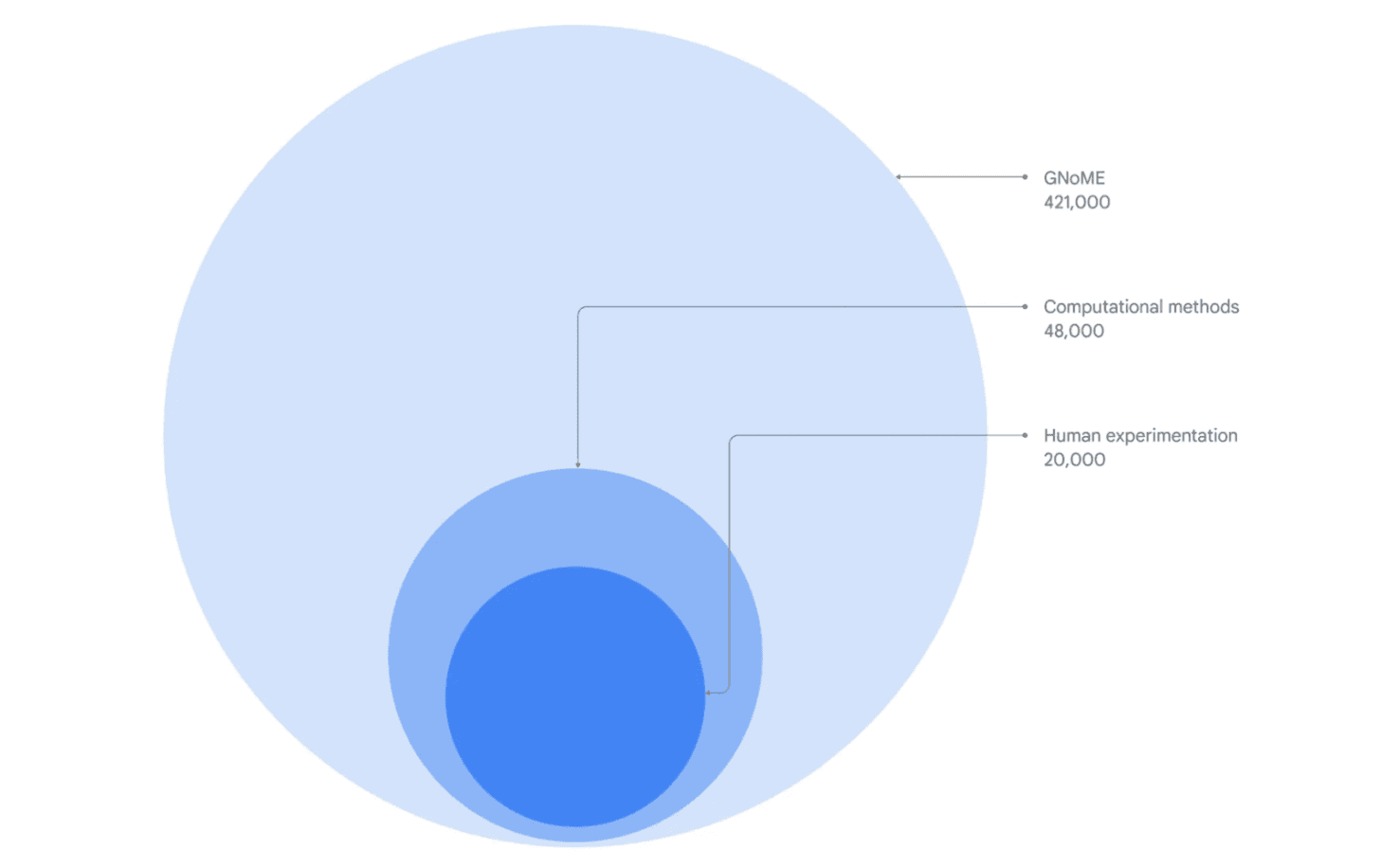
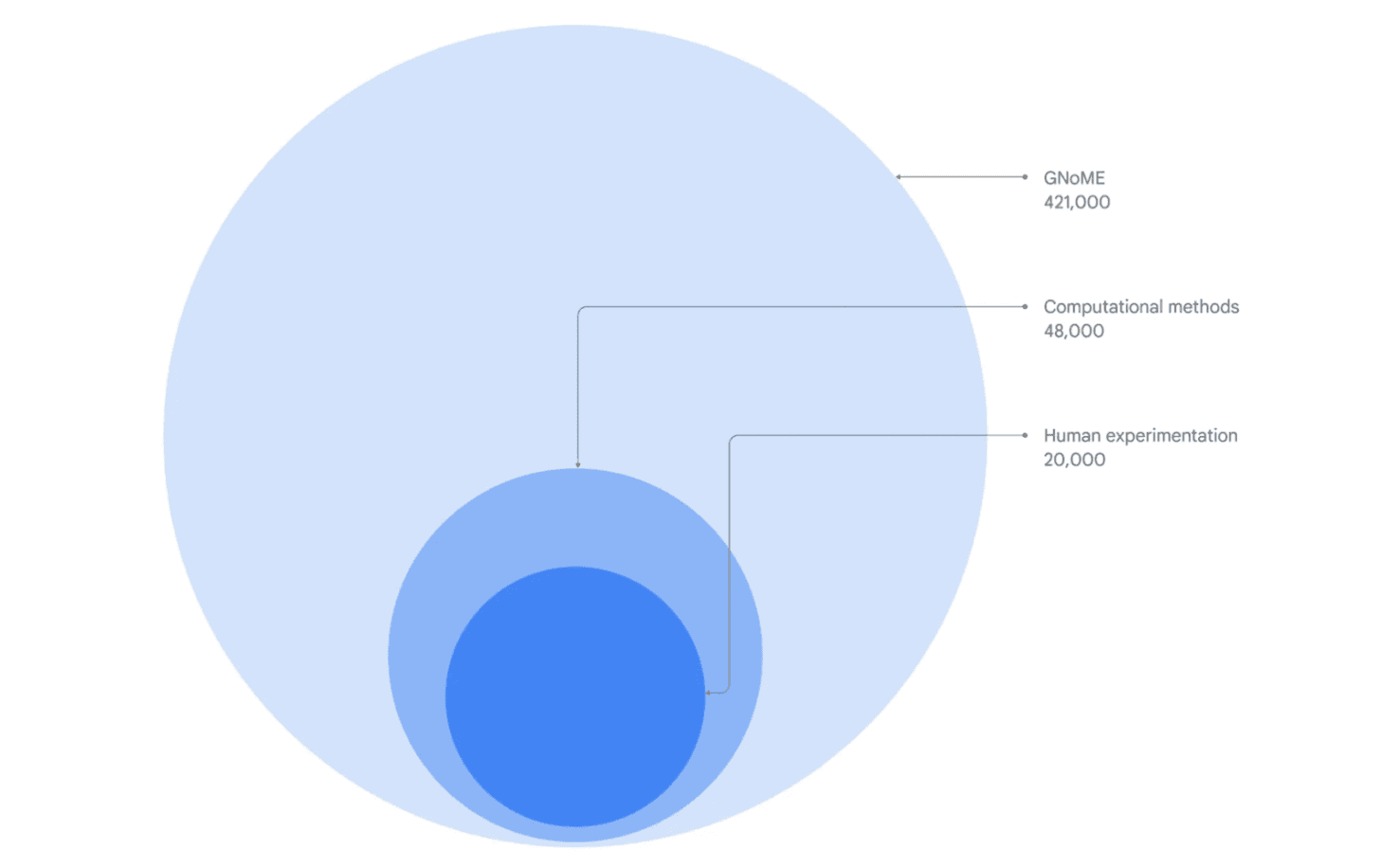
What are the results?
These results enabled to synthesize real materials in 17 days of closed-loop operations. Indeed, Berkeley’s A-Lab performed 355 experiments and successfully synthesized 41 novel compounds from a set of 58 novel inorganic crystal solid targets. This tool now enabled to predict stability with more accuracy and experimental viability, replacing the trial and error only method.
What is the outcome?
These results enabled to synthesize real materials in 17 days of closed-loop operations. Indeed, Berkeley’s A-Lab performed 355 experiments and successfully synthesized 41 novel compounds from a set of 58 novel inorganic crystal solid targets. This tool now enabled to predict stability with more accuracy and experimental viability, replacing the trial and error only method.
What is the outcome?
These results enabled to synthesize real materials in 17 days of closed-loop operations. Indeed, Berkeley’s A-Lab performed 355 experiments and successfully synthesized 41 novel compounds from a set of 58 novel inorganic crystal solid targets. This tool now enabled to predict stability with more accuracy and experimental viability, replacing the trial and error only method.
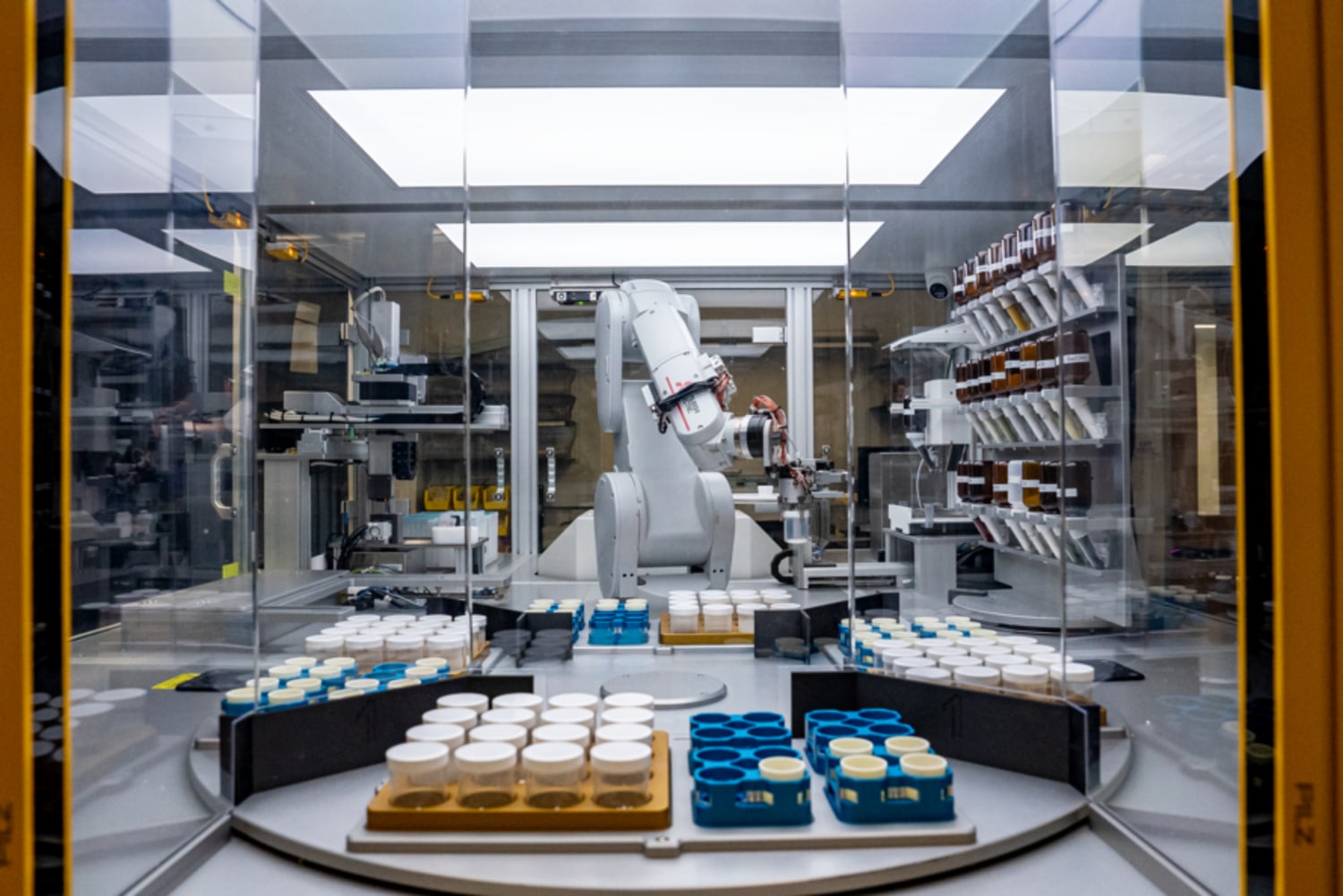
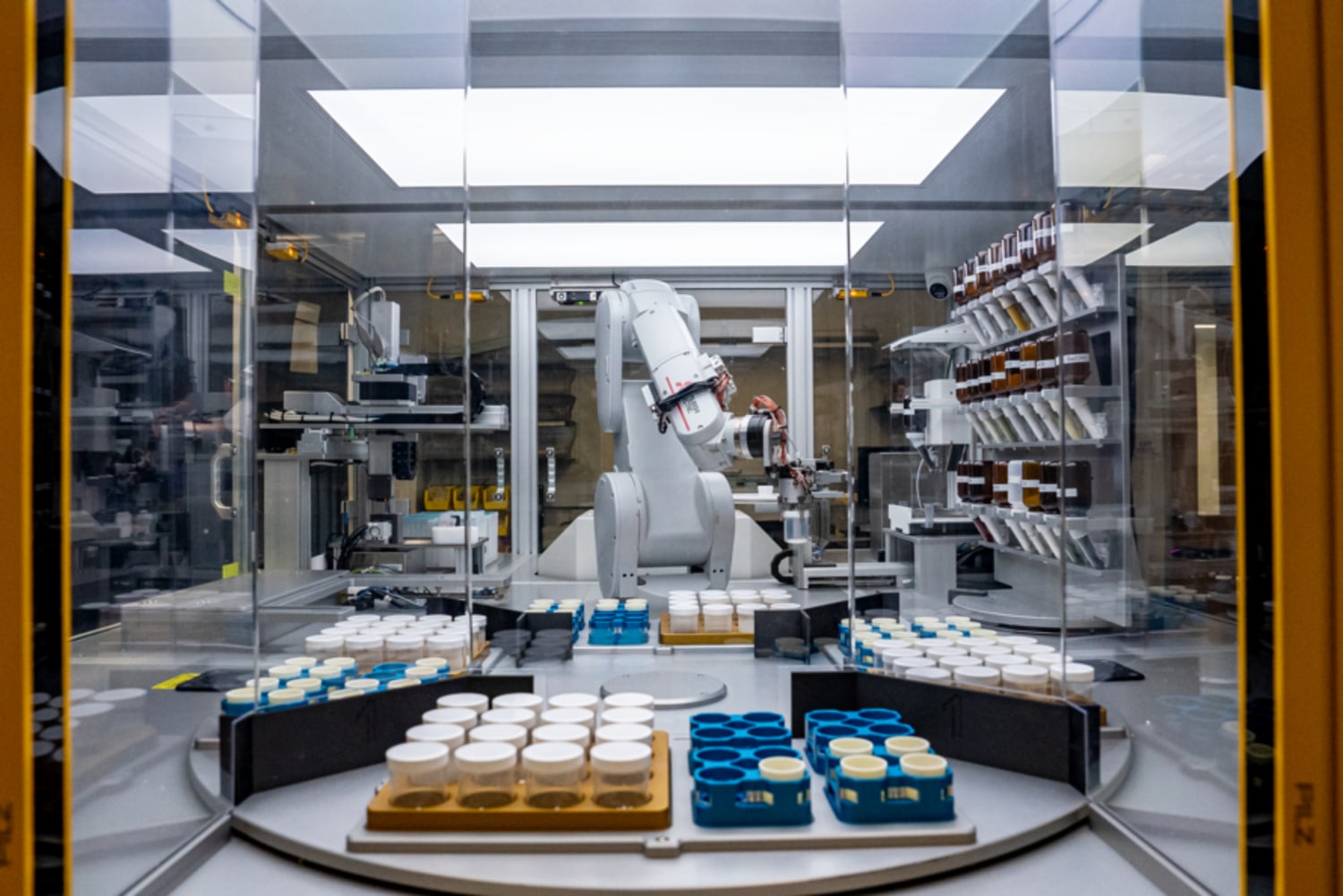
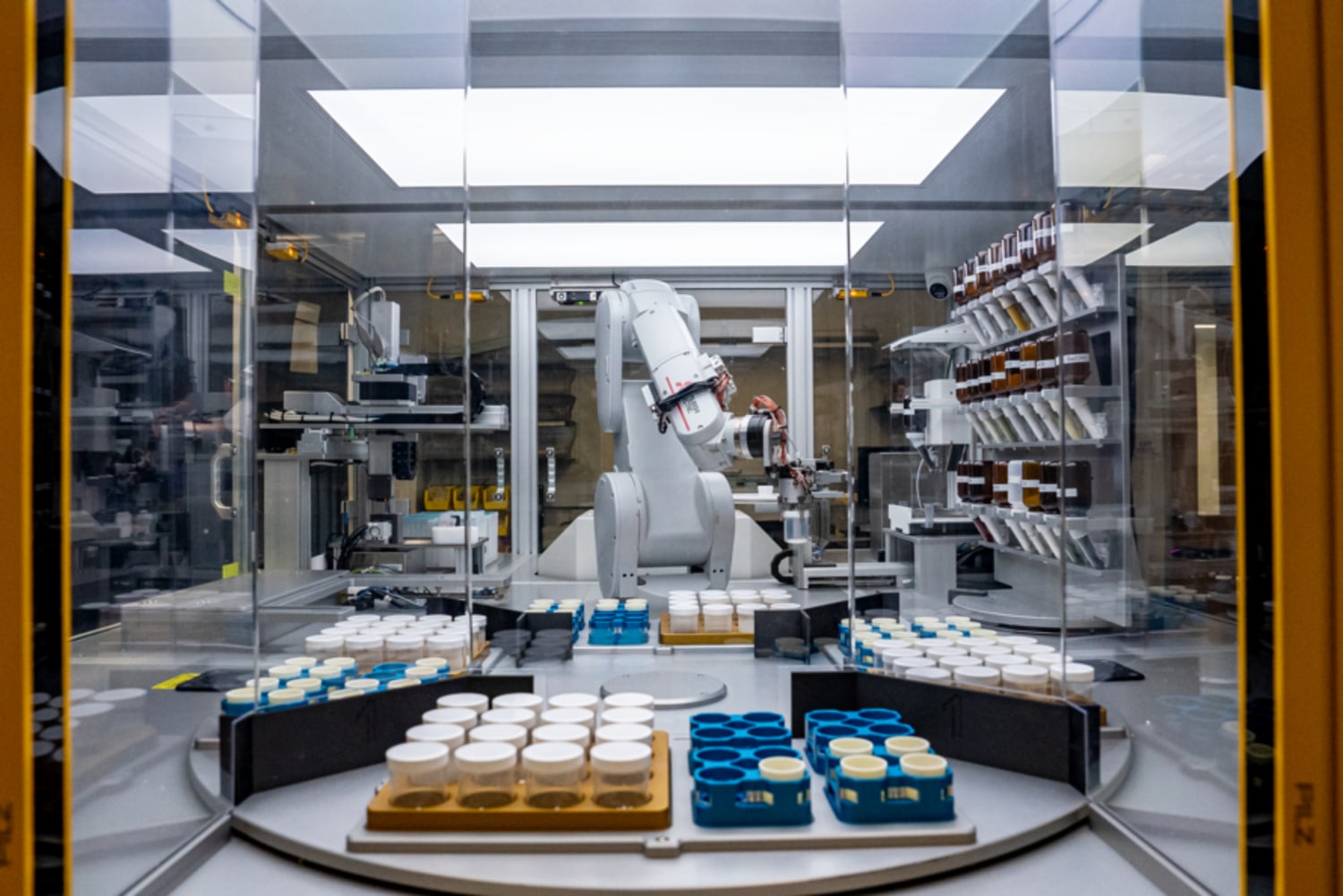
What are the applications?
Inorganic crystals have a wide array of applications across various fields due to their unique properties, such as high thermal stability, electrical conductivity, and mechanical strength. Hence it has applications in various fields such as microchips, batteries, optics and photovoltaics.
What are the applications?
Inorganic crystals have a wide array of applications across various fields due to their unique properties, such as high thermal stability, electrical conductivity, and mechanical strength. Hence it has applications in various fields such as microchips, batteries, optics and photovoltaics.
What are the applications?
Inorganic crystals have a wide array of applications across various fields due to their unique properties, such as high thermal stability, electrical conductivity, and mechanical strength. Hence it has applications in various fields such as microchips, batteries, optics and photovoltaics.
B. Zoom on their AI-driven approach
B. Zoom on their AI-driven approach
What does their technology look like?
The technology developed in the paper revolves around a machine learning-driven approach to materials discovery, with a particular focus on using active learning and deep learning models to predict and explore new materials structures. The model utilizes a foundation model trained on a large dataset, which is further refined through iterations involving a Graph Neural Network (GNN) to predict the stability of materials.
Additionally, the technology incorporates a workflow for active learning, where six rounds of data selection are employed to progressively improve the model's accuracy. Furthermore, AIRSS (Ab Initio Random Structure Searching) is used to convert 2D materials candidates into multiple 3D structures. These 3D structures undergo quantum simulations, specifically using density functional theory (DFT), to assess their stability. Structures meeting the stability criteria are then added to the database.
What is noteworthy is the integration of AI at various stages of the workflow, as well as its combination with simulations approach.
What is their technology?
The technology developed in the paper revolves around a machine learning-driven approach to materials discovery, with a particular focus on using active learning and deep learning models to predict and explore new materials structures. The model utilizes a foundation model trained on a large dataset, which is further refined through iterations involving a Graph Neural Network (GNN) to predict the stability of materials.
Additionally, the technology incorporates a workflow for active learning, where six rounds of data selection are employed to progressively improve the model's accuracy. Furthermore, AIRSS (Ab Initio Random Structure Searching) is used to convert 2D materials candidates into multiple 3D structures. These 3D structures undergo quantum simulations, specifically using density functional theory (DFT), to assess their stability. Structures meeting the stability criteria are then added to the database.
What is noteworthy is the integration of AI at various stages of the workflow, as well as its combination with simulations approach.
What does their technology look like?
The technology developed in the paper revolves around a machine learning-driven approach to materials discovery, with a particular focus on using active learning and deep learning models to predict and explore new materials structures. The model utilizes a foundation model trained on a large dataset, which is further refined through iterations involving a Graph Neural Network (GNN) to predict the stability of materials.
Additionally, the technology incorporates a workflow for active learning, where six rounds of data selection are employed to progressively improve the model's accuracy. Furthermore, AIRSS (Ab Initio Random Structure Searching) is used to convert 2D materials candidates into multiple 3D structures. These 3D structures undergo quantum simulations, specifically using density functional theory (DFT), to assess their stability. Structures meeting the stability criteria are then added to the database.
What is noteworthy is the integration of AI at various stages of the workflow, as well as its combination with simulations approach.
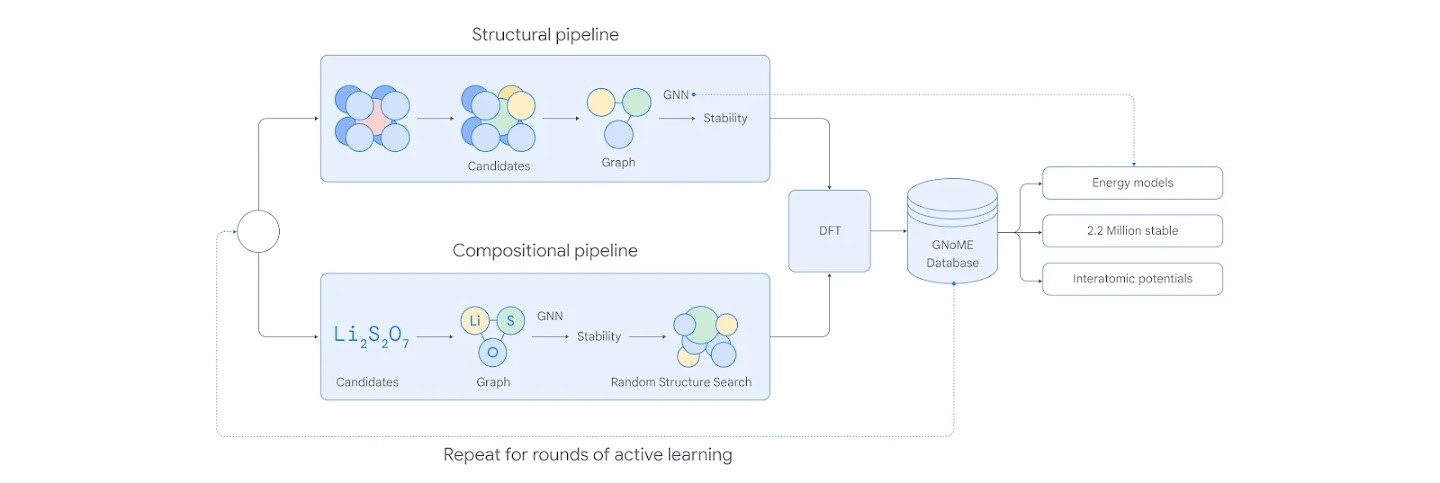
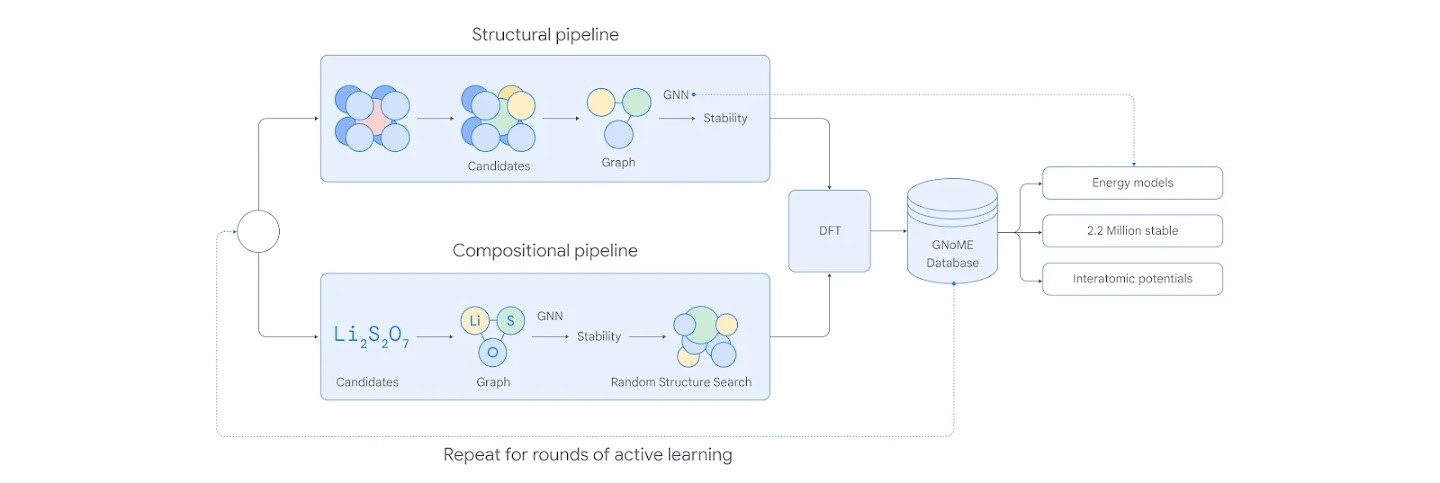
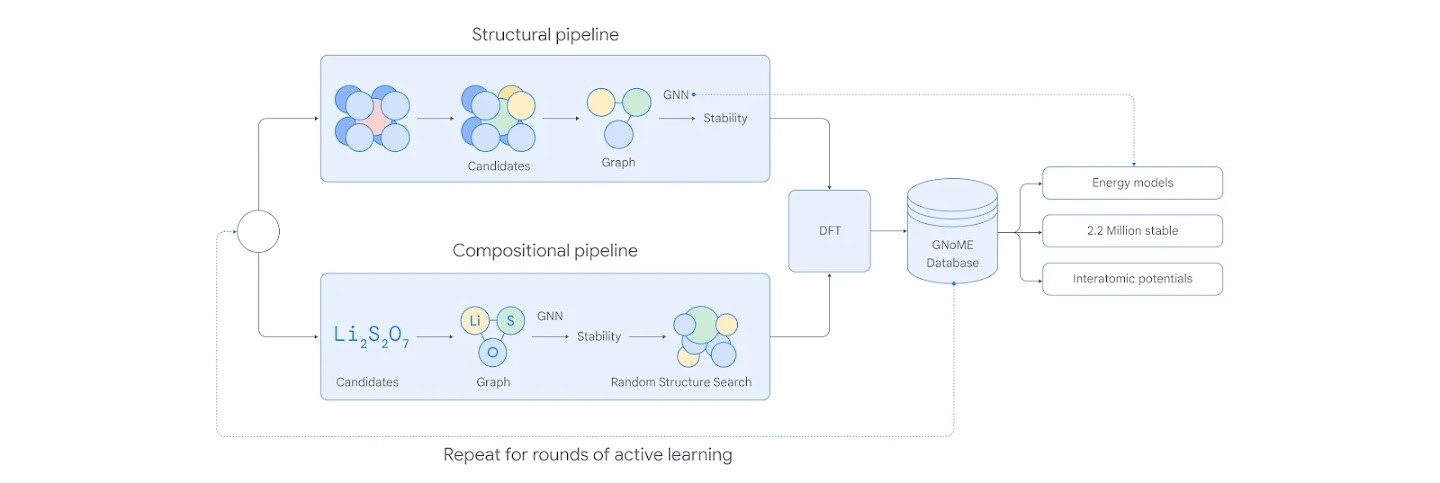
What is the architecture of the AI model?
The architecture of the model is centered around a Graph Neural Networks (GNN) that was iteratively trained on data selected through an active learning process. This model incorporates deep ensembles, which are employed to quantify uncertainty and guide the active learning process. The active learning strategy involves selecting the most uncertain predictions for additional labeling and retraining, thereby improving the model's robustness and accuracy.
Besides, the zero-shot generalization enables Google Deepmind to predict the stability of new structures not present in the database. Hence, they are able to generalize and extrapolate outside the domain to find new structures.
Additionally, the architecture incorporates a temporal component to account for dynamic cases, integrating molecular dynamic simulations to ensure that the model can handle time-dependent aspects of materials behavior.
To conclude, the overall workflow involves multiple pipelines: generating candidate structures, evaluating their stability using DFT simulations, and refining the model iteratively.
Limitations of the paper regarding real-world applications
While the paper presents significant advancements in materials discovery using AI, it has several limitations. One notable limitation is its lack of focus on complex materials, particularly those with high stoichiometric numbers. These materials, which are common in industrial applications (e.g., alloys with composition accuracy close to 1% or lower), require larger graphs for modeling, which in turn demand more computational resources and time when using DFT calculations. This complexity is critical for real-world applications, yet it is not adequately addressed in the paper.
Additionally, the concept of stability, while crucial, is explored in isolation without sufficient consideration of other important factors such as processability, synthesizability, costs, time of development, which are essential for practical industrial materials development.
Besides, their model only provides a list of new materials regardless of their properties, which is in the end what matters most for industrials. Last, they trained their model on a large data set collected thanks to the Materials Project. In reality, industrials struggle to structure the data from their experiments and hardly come up with more than a few hundreds data points.
To conclude, while the integration of AI and simulation marks a breakthrough, the pipeline's complexity and the scalability of the approach for industrial-scale problems remain underexplored. GNoME does not tackle the misalignment of academic research and industrial innovation, only focusing on the discovery approach.
What is the architecture of the model?
The architecture of the model is centered around a Graph Neural Networks (GNN) that was iteratively trained on data selected through an active learning process. This model incorporates deep ensembles, which are employed to quantify uncertainty and guide the active learning process. The active learning strategy involves selecting the most uncertain predictions for additional labeling and retraining, thereby improving the model's robustness and accuracy.
Besides, the zero-shot generalization enables Google Deepmind to predict the stability of new structures not present in the database. Hence, they are able to generalize and extrapolate outside the domain to find new structures.
Additionally, the architecture incorporates a temporal component to account for dynamic cases, integrating molecular dynamic simulations to ensure that the model can handle time-dependent aspects of materials behavior.
To conclude, the overall workflow involves multiple pipelines: generating candidate structures, evaluating their stability using DFT simulations, and refining the model iteratively.
Limitations of the paper regarding real-world applications
While the paper presents significant advancements in materials discovery using AI, it has several limitations. One notable limitation is its lack of focus on complex materials, particularly those with high stoichiometric numbers. These materials, which are common in industrial applications (e.g., alloys with composition accuracy close to 1% or lower), require larger graphs for modeling, which in turn demand more computational resources and time when using DFT calculations. This complexity is critical for real-world applications, yet it is not adequately addressed in the paper.
Additionally, the concept of stability, while crucial, is explored in isolation without sufficient consideration of other important factors such as processability, synthesizability, costs, time of development, which are essential for practical industrial materials development.
Besides, their model only provides a list of new materials regardless of their properties, which is in the end what matters most for industrials. Last, they trained their model on a large data set collected thanks to the Materials Project. In reality, industrials struggle to structure the data from their experiments and hardly come up with more than a few hundreds data points.
To conclude, while the integration of AI and simulation marks a breakthrough, the pipeline's complexity and the scalability of the approach for industrial-scale problems remain underexplored. GNoME does not tackle the misalignment of academic research and industrial innovation, only focusing on the discovery approach.
What is the architecture of the model?
The architecture of the model is centered around a Graph Neural Networks (GNN) that was iteratively trained on data selected through an active learning process. This model incorporates deep ensembles, which are employed to quantify uncertainty and guide the active learning process. The active learning strategy involves selecting the most uncertain predictions for additional labeling and retraining, thereby improving the model's robustness and accuracy.
Besides, the zero-shot generalization enables Google Deepmind to predict the stability of new structures not present in the database. Hence, they are able to generalize and extrapolate outside the domain to find new structures.
Additionally, the architecture incorporates a temporal component to account for dynamic cases, integrating molecular dynamic simulations to ensure that the model can handle time-dependent aspects of materials behavior.
To conclude, the overall workflow involves multiple pipelines: generating candidate structures, evaluating their stability using DFT simulations, and refining the model iteratively.
Limitations of the paper regarding real-world applications
While the paper presents significant advancements in materials discovery using AI, it has several limitations. One notable limitation is its lack of focus on complex materials, particularly those with high stoichiometric numbers. These materials, which are common in industrial applications (e.g., alloys with composition accuracy close to 1% or lower), require larger graphs for modeling, which in turn demand more computational resources and time when using DFT calculations. This complexity is critical for real-world applications, yet it is not adequately addressed in the paper.
Additionally, the concept of stability, while crucial, is explored in isolation without sufficient consideration of other important factors such as processability, synthesizability, costs, time of development, which are essential for practical industrial materials development.
Besides, their model only provides a list of new materials regardless of their properties, which is in the end what matters most for industrials. Last, they trained their model on a large data set collected thanks to the Materials Project. In reality, industrials struggle to structure the data from their experiments and hardly come up with more than a few hundreds data points.
To conclude, while the integration of AI and simulation marks a breakthrough, the pipeline's complexity and the scalability of the approach for industrial-scale problems remain underexplored. GNoME does not tackle the misalignment of academic research and industrial innovation, only focusing on the discovery approach.
C. Race launched to discover new materials using AI
C. Race launched to discover new materials using AI
C. Race launched to discover new materials using AI
GNoME paper catalyzed the discussions around how AI can help discover new stable materials relevant to industry use cases. AI has significantly transformed various scientific fields in recent years, such as drug discovery. However, advancements in materials development have been very limited until recently.
At Osium AI, we identified this gap and therefore developed our proprietary AI technology, actually way before Google DeepMind published their initial research on the topic. Consequently, it was exciting for us to witness major technology companies releasing their own AI-driven materials science research several months later and therefore fostering great discussions on the impact of AI on the materials science field.
However, many challenges remain that must be addressed to enable AI to drastically transform the field of materials science: how to help industrials better leverage their data, how to integrate this technology into their workflow and how to enable this technology to actually fit the needs of industrials… Finding solutions to these challenges is crucial for enabling industry leaders to transform their R&D workflows through AI.
This is where Osium AI comes into play.
GNoME paper catalyzed the discussions around how AI can help discover new stable materials relevant to industry use cases. AI has significantly transformed various scientific fields in recent years, such as drug discovery. However, advancements in materials development have been very limited until recently.
At Osium AI, we identified this gap and therefore developed our proprietary AI technology, actually way before Google DeepMind published their initial research on the topic. Consequently, it was exciting for us to witness major technology companies releasing their own AI-driven materials science research several months later and therefore fostering great discussions on the impact of AI on the materials science field.
However, many challenges remain that must be addressed to enable AI to drastically transform the field of materials science: how to help industrials better leverage their data, how to integrate this technology into their workflow and how to enable this technology to actually fit the needs of industrials… Finding solutions to these challenges is crucial for enabling industry leaders to transform their R&D workflows through AI.
This is where Osium AI comes into play.
GNoME paper catalyzed the discussions around how AI can help discover new stable materials relevant to industry use cases. AI has significantly transformed various scientific fields in recent years, such as drug discovery. However, advancements in materials development have been very limited until recently.
At Osium AI, we identified this gap and therefore developed our proprietary AI technology, actually way before Google DeepMind published their initial research on the topic. Consequently, it was exciting for us to witness major technology companies releasing their own AI-driven materials science research several months later and therefore fostering great discussions on the impact of AI on the materials science field.
However, many challenges remain that must be addressed to enable AI to drastically transform the field of materials science: how to help industrials better leverage their data, how to integrate this technology into their workflow and how to enable this technology to actually fit the needs of industrials… Finding solutions to these challenges is crucial for enabling industry leaders to transform their R&D workflows through AI.
This is where Osium AI comes into play.
II. Osium AI’s industry-oriented platform
II. Osium AI’s industry-oriented platform
II. Osium AI’s industry-oriented platform
Our vision
Our vision
Our vision
Value lies in the understanding of industrial needs
Osium AI aims at bridging the gap between research and industry. To do so, Osium AI positions as a leader in materials discovery powered by AI and provides a solution to all industrials tailored to their needs, their use cases and their data. At Osium AI, we developed a proprietary AI technology to leverage the data of industrials and accelerate their R&D phases from discovery to scaling up the development of their materials and chemicals. We put a strong emphasis on understanding the needs of our customers. Therefore, we built our product to fulfill the industry constraints and needs of our customers.
Osium provides an AI-powered platform specialized in materials and chemicals R&D
We observed many times that industrials want to be more efficient with their R&D processes. They are looking for ways to leverage the data that they generate. This is why Osium AI positions as an AI-powered platform enabling industrials to improve their R&D by easily benefiting from the power of AI. Empowering our customers to integrate AI into the whole value chain of their materials and chemicals development is our priority. We help them accelerate, they innovate.
Value lies in the understanding of industrial needs
Osium AI aims at bridging the gap between stakeholders. To do so, Osium AI positions as a leader in materials discovery powered by AI and provides a solution to all industrials tailored to their needs, their use case and their data. At Osium AI, we developed a proprietary AI technology to leverage the data of industrials and accelerate their R&D phases from discovery to scaling up the development of their materials and chemicals. We put a strong emphasis on understanding the needs of our customers. Therefore, we built our product based on the constraints and needs of clients.
Osium is a solution provider
We have the firm conviction that industrials want to internalize their R&D processes. Indeed, they want to leverage the data that they generate from their experiments and keep full ownership of their findings. This is why Osium AI positions as a solution provider, enabling industrials to internalize their R&D while easily benefiting from the power of AI. Empowering our customers to control and own all the value chain of their materials and chemicals development is our priority. We help them accelerate, they innovate.
Value lies in the understanding of industrial needs
Osium AI aims at bridging the gap between stakeholders. To do so, Osium AI positions as a leader in materials discovery powered by AI and provides a solution to all industrials tailored to their needs, their use case and their data. At Osium AI, we developed a proprietary AI technology to leverage the data of industrials and accelerate their R&D phases from discovery to scaling up the development of their materials and chemicals. We put a strong emphasis on understanding the needs of our customers. Therefore, we built our product based on the constraints and needs of clients.
Osium is a solution provider
We have the firm conviction that industrials want to internalize their R&D processes. Indeed, they want to leverage the data that they generate from their experiments and keep full ownership of their findings. This is why Osium AI positions as a solution provider, enabling industrials to internalize their R&D while easily benefiting from the power of AI. Empowering our customers to control and own all the value chain of their materials and chemicals development is our priority. We help them accelerate, they innovate.
B. Our Solution
B. Our Solution
B. Our Solution
Driving the most value
In order to drive the most value for our customers, we built the features enabling industrials to speed up their materials R&D while taking into account their real industry constraints. Our model empowers our customers with a smart selection of experiments to conduct. We know how expensive each experience is. Hence, it is crucial to guide our customers towards the experiments which have the highest chances to succeed. Our solution takes into account real-world industrial constraints.
In particular, two features are coupled together to enable our customers to derive the most value for their R&D. The first one is the properties prediction feature. This feature takes the material characteristics as input and predicts the properties of this material. Then the materials design feature enables chemists to generate optimal materials and chemicals candidates from their specifications, reducing the need for physical testing and therefore the overall time for materials development.
Flexible solution tailor-made solution for industrials
In addition, our solutions can adapt quickly to the needs of the clients depending on their specific use case, data available or the output sought. Our solutions enable our customers to dwindle candidates of materials as their constraints grow and integrate evolving real-world constraints (e.g. processing constraints, costs, sustainability requirements). The end goal for industrials is to limit the time and cost of R&D, therefore precise identification of good candidates which can be processed and synthesized is essential to limit the physical testing phase. Considering that constraints and specifications can change rapidly, the ability to adapt swiftly is essential for efficient R&D processes. Thus, the flexibility of our platform is crucial.
Seamless integration
Besides, we strive in building the most intuitive solution to integrate effortlessly into our customers’ workflow. Our platform was built by scientists for scientists, without any AI prerequisite for the users.
Driving the most value
In order to drive the most value for our customers, we built the features enabling industrials to speed up their materials R&D while taking into account their real industry constraints. Our model empowers our customers with a smart selection of experiments to conduct. We know how expensive each experience is. Hence, it is crucial to guide our customers towards the experiments which have the highest chances to succeed. Our solution takes into account real-world industrial constraints.
In particular, two features are coupled together to enable our customers to derive the most value for their R&D. The first one is the properties prediction feature. This feature takes the material characteristics as input and predicts the properties of this material. Then the materials design feature enables chemists to generate optimal materials and chemicals candidates from their specifications, reducing the need for physical testing and therefore the overall time for materials development.
Flexible solution tailor-made solution for industrials
In addition, our solutions can adapt quickly to the needs of the clients depending on their specific use case, data available or the output sought. Our solutions enable our customers to dwindle candidates of materials as their constraints grow and integrate evolving real-world constraints (e.g. processing constraints, costs, sustainability requirements). The end goal for industrials is to limit the time and cost of R&D, therefore precise identification of good candidates which can be processed and synthesized is essential to limit the physical testing phase. Considering that constraints and specifications can change rapidly, the ability to adapt swiftly is essential for efficient R&D processes. Thus, the flexibility of our platform is crucial.
Seamless integration
Besides, we strive in building the most intuitive solution to integrate effortlessly into our customers’ workflow. Our platform was built by scientists for scientists, without any AI prerequisite for the users.
Driving the most value
In order to drive the most value for our customers, we built the features enabling industrials to speed up their materials R&D while taking into account their real industry constraints. Our model empowers our customers with a smart selection of experiments to conduct. We know how expensive each experience is. Hence, it is crucial to guide our customers towards the experiments which have the highest chances to succeed. Our solution takes into account real-world industrial constraints.
In particular, two features are coupled together to enable our customers to derive the most value for their R&D. The first one is the properties prediction feature. This feature takes the material characteristics as input and predicts the properties of this material. Then the materials design feature enables chemists to generate optimal materials and chemicals candidates from their specifications, reducing the need for physical testing and therefore the overall time for materials development.
Flexible solution tailor-made solution for industrials
In addition, our solutions can adapt quickly to the needs of the clients depending on their specific use case, data available or the output sought. Our solutions enable our customers to dwindle candidates of materials as their constraints grow and integrate evolving real-world constraints (e.g. processing constraints, costs, sustainability requirements). The end goal for industrials is to limit the time and cost of R&D, therefore precise identification of good candidates which can be processed and synthesized is essential to limit the physical testing phase. Considering that constraints and specifications can change rapidly, the ability to adapt swiftly is essential for efficient R&D processes. Thus, the flexibility of our platform is crucial.
Seamless integration
Besides, we strive in building the most intuitive solution to integrate effortlessly into our customers’ workflow. Our platform was built by scientists for scientists, without any AI prerequisite for the users.
C. Our technology
C. Our technology
C. Our technology
Physics-informed ML
To develop our product, we benefited from the solid experience of our founding team having worked at Google X and released several patents in AI already. We built our proprietary machine learning models to provide the most accurate predictions, especially proprietary models at the intersection of AI and physical laws. Our unique approach enables us to model the complex behaviors of materials.
Robustness to small training databases
Besides, we strived to create a model able to provide accurate predictions without needing a lot of data points for training. We derive value even out of small datasets. We comply with the real world challenges of our customers as they struggle to structure their data, needing a flexible and robust solution like Osium AI.
Data privacy
Furthermore, our platform complies with the highest data security standards. Being able to secure the data from end to end, giving our customers full ownership of their data and enabling them to easily leverage the results they generate thanks to our solution is our priority. We know how valuable data is.
Physics-informed ML
To develop our product, we benefited from the solid experience of our founding team having worked at Google X and released several patents in AI already. We built our proprietary machine learning models to provide the most accurate predictions, especially proprietary models at the intersection of AI and physical laws. Our unique approach enables us to model the complex behaviors of materials.
Robustness to small training databases
Besides, we strived to create a model able to provide accurate predictions without needing a lot of data points for training. We derive value even out of small datasets. We comply with the real world challenges of our customers as they struggle to structure their data, needing a flexible and robust solution like Osium AI.
Data privacy
Furthermore, our platform complies with the highest data security standards. Being able to secure the data from end to end, giving our customers full ownership of their data and enabling them to easily leverage the results they generate thanks to our solution is our priority. We know how valuable data is.
Physics-informed ML
To develop our product, we benefited from the solid experience of our founding team having worked at Google X and released several patents in AI already. We built our proprietary machine learning models to provide the most accurate predictions, especially proprietary models at the intersection of AI and physical laws. Our unique approach enables us to model the complex behaviors of materials.
Robustness to small training databases
Besides, we strived to create a model able to provide accurate predictions without needing a lot of data points for training. We derive value even out of small datasets. We comply with the real world challenges of our customers as they struggle to structure their data, needing a flexible and robust solution like Osium AI.
Data privacy
Furthermore, our platform complies with the highest data security standards. Being able to secure the data from end to end, giving our customers full ownership of their data and enabling them to easily leverage the results they generate thanks to our solution is our priority. We know how valuable data is.
Conclusion
Conclusion
Conclusion
GNoME represents a significant breakthrough in the field of materials discovery, showcasing the potential of AI-driven innovation to revolutionize the way new materials are developed. Advanced AI techniques open up new possibilities for innovation in the materials and chemicals field: optimize processes, provide reliable properties prediction and extract valuable insights from unstructured data.
GNoME’s research paper shed light on the significant impact AI can have on materials development. However, GNoME's current framework does not fully account for the practical constraints faced by industries, highlighting a key limitation in the paper. The gap between academic research and industrial application remains significant, as research does not usually take into account challenges and constraints that industries face.
Osium AI addresses these challenges by bridging the gap between research and industrial innovation. As a pioneering solution provider of an AI-powered platform tailored for materials science and built on proprietary technology, Osium AI not only understands industrial constraints but also effectively incorporates them into its AI-driven materials development platform. Furthermore, Osium AI’s platform seamlessly integrates into the entire value chain of industrial R&D workflows, easily bringing its latest AI advancements to the materials industry.
GNoME represents a significant breakthrough in the field of materials discovery, showcasing the potential of AI-driven innovation to revolutionize the way new materials are developed. Advanced AI techniques open up new possibilities for innovation in the materials and chemicals field: optimize processes, provide reliable properties prediction and extract valuable insights from unstructured data.
GNoME’s research paper shed light on the significant impact AI can have on materials development. However, GNoME's current framework does not fully account for the practical constraints faced by industries, highlighting a key limitation in the paper. The gap between academic research and industrial application remains significant, as research does not usually take into account challenges and constraints that industries face.
Osium AI addresses these challenges by bridging the gap between research and industrial innovation. As a pioneering solution provider of an AI-powered platform tailored for materials science and built on proprietary technology, Osium AI not only understands industrial constraints but also effectively incorporates them into its AI-driven materials development platform. Furthermore, Osium AI’s platform seamlessly integrates into the entire value chain of industrial R&D workflows, easily bringing its latest AI advancements to the materials industry.
GNoME represents a significant breakthrough in the field of materials discovery, showcasing the potential of AI-driven innovation to revolutionize the way new materials are developed. Advanced AI techniques open up new possibilities for innovation in the materials and chemicals field: optimize processes, provide reliable properties prediction and extract valuable insights from unstructured data.
GNoME’s research paper shed light on the significant impact AI can have on materials development. However, GNoME's current framework does not fully account for the practical constraints faced by industries, highlighting a key limitation in the paper. The gap between academic research and industrial application remains significant, as research does not usually take into account challenges and constraints that industries face.
Osium AI addresses these challenges by bridging the gap between research and industrial innovation. As a pioneering solution provider of an AI-powered platform tailored for materials science and built on proprietary technology, Osium AI not only understands industrial constraints but also effectively incorporates them into its AI-driven materials development platform. Furthermore, Osium AI’s platform seamlessly integrates into the entire value chain of industrial R&D workflows, easily bringing its latest AI advancements to the materials industry.